Поиск :
Личный кабинет :
Электронный каталог: Marin, E. - Regression-Based Active Learning for Accessible Acceleration of Ultra-Large Library Docking
Marin, E. - Regression-Based Active Learning for Accessible Acceleration of Ultra-Large Library Docking
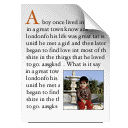
Статья
Автор: Marin, E.
Journal of Chemical Information and Modeling [Electronic resource]: Regression-Based Active Learning for Accessible Acceleration of Ultra-Large Library Docking
б.г.
ISBN отсутствует
Автор: Marin, E.
Journal of Chemical Information and Modeling [Electronic resource]: Regression-Based Active Learning for Accessible Acceleration of Ultra-Large Library Docking
б.г.
ISBN отсутствует
Статья
Marin, E.
Regression-Based Active Learning for Accessible Acceleration of Ultra-Large Library Docking / E.Marin, A.Rogachev, V.Borshchevskiy, [a.o.] // Journal of Chemical Information and Modeling [Electronic resource]. – 2024. – Vol. 64, No. 7. – P. 2612-2623. – URL: https://doi.org/10.1021/acs.jcim.3c01661. – Bibliogr.: P.2622-2623.
Structure-based drug discovery is a process for both hit finding and optimization that relies on a validated three-dimensional model of a target biomolecule, used to rationalize the structure–function relationship for this particular target. An ultralarge virtual screening approach has emerged recently for rapid discovery of high-affinity hit compounds, but it requires substantial computational resources. This study shows that active learning with simple linear regression models can accelerate virtual screening, retrieving up to 90% of the top-1% of the docking hit list after docking just 10% of the ligands. The results demonstrate that it is unnecessary to use complex models, such as deep learning approaches, to predict the imprecise results of ligand docking with a low sampling depth. Furthermore, we explore active learning meta-parameters and find that constant batch size models with a simple ensembling method provide the best ligand retrieval rate. Finally, our approach is validated on the ultralarge size virtual screening data set, retrieving 70% of the top-0.05% of ligands after screening only 2% of the library. Altogether, this work provides a computationally accessible approach for accelerated virtual screening that can serve as a blueprint for the future design of low-compute agents for exploration of the chemical space via large-scale accelerated docking. With recent breakthroughs in protein structure prediction, this method can significantly increase accessibility for the academic community and aid in the rapid discovery of high-affinity hit compounds for various targets.
ОИЯИ = ОИЯИ (JINR)2024
Спец.(статьи,препринты) = С 44 - Аналитическая химия
Спец.(статьи,препринты) = Ц 849 - Искусственный интеллект. Теория и практика
Спец.(статьи,препринты) = Ц 840 г1 - Банки и базы данных
Бюллетени = 50/024
Marin, E.
Regression-Based Active Learning for Accessible Acceleration of Ultra-Large Library Docking / E.Marin, A.Rogachev, V.Borshchevskiy, [a.o.] // Journal of Chemical Information and Modeling [Electronic resource]. – 2024. – Vol. 64, No. 7. – P. 2612-2623. – URL: https://doi.org/10.1021/acs.jcim.3c01661. – Bibliogr.: P.2622-2623.
Structure-based drug discovery is a process for both hit finding and optimization that relies on a validated three-dimensional model of a target biomolecule, used to rationalize the structure–function relationship for this particular target. An ultralarge virtual screening approach has emerged recently for rapid discovery of high-affinity hit compounds, but it requires substantial computational resources. This study shows that active learning with simple linear regression models can accelerate virtual screening, retrieving up to 90% of the top-1% of the docking hit list after docking just 10% of the ligands. The results demonstrate that it is unnecessary to use complex models, such as deep learning approaches, to predict the imprecise results of ligand docking with a low sampling depth. Furthermore, we explore active learning meta-parameters and find that constant batch size models with a simple ensembling method provide the best ligand retrieval rate. Finally, our approach is validated on the ultralarge size virtual screening data set, retrieving 70% of the top-0.05% of ligands after screening only 2% of the library. Altogether, this work provides a computationally accessible approach for accelerated virtual screening that can serve as a blueprint for the future design of low-compute agents for exploration of the chemical space via large-scale accelerated docking. With recent breakthroughs in protein structure prediction, this method can significantly increase accessibility for the academic community and aid in the rapid discovery of high-affinity hit compounds for various targets.
ОИЯИ = ОИЯИ (JINR)2024
Спец.(статьи,препринты) = С 44 - Аналитическая химия
Спец.(статьи,препринты) = Ц 849 - Искусственный интеллект. Теория и практика
Спец.(статьи,препринты) = Ц 840 г1 - Банки и базы данных
Бюллетени = 50/024