Поиск :
Личный кабинет :
Электронный каталог: Uzhinskiy, A. - Efficient Pipeline for Plant Disease Classification
Uzhinskiy, A. - Efficient Pipeline for Plant Disease Classification
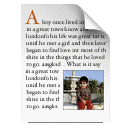
Статья
Автор: Uzhinskiy, A.
Natural Science Review: Efficient Pipeline for Plant Disease Classification
б.г.
ISBN отсутствует
Автор: Uzhinskiy, A.
Natural Science Review: Efficient Pipeline for Plant Disease Classification
б.г.
ISBN отсутствует
Статья
Uzhinskiy, A.
Efficient Pipeline for Plant Disease Classification / A.Uzhinskiy. – Text: electronic // Natural Science Review. – 2025. – Vol. 2, No. 2. – P. 100201. – URL: https://nsr-jinr.ru/index.php/nsr/article/view/32/29. – Bibliogr.: 28.
Accurate identification of disease and correct treatment policy can save and increase yield. Differentdeep learning methods have emerged as an effective solution to this problem. Still, the challenges posedby limited datasets and the similarities in disease symptoms make traditional methods, such as transferlearning from models pre-trained on large-scale datasets like ImageNet, less effective. In this study, aself-collected dataset from the DoctorP project, consisting of 46 distinct classes and 2615 images, wasutilized. DoctorP is a multifunctional platform for plant disease detection oriented on agricultural andornamental crop. The platform has different interfaces like mobile applications for iOS and Android,a Telegram bot, and an API for external services. Users and services send photos of the diseasedplants in to the platform and can get prediction and treatment recommendation for their case. Theplatform supports a wide range of disease classification models. MobileNet_v2 and a Triplet lossfunction were previously used to create models. Extensive increase in the number of disease classesforces new experiment with architectures and training approaches. In the current research, an effectivesolution based on ConvNeXt architecture and Large Margin Cosine Loss is proposed to classify 46different plant diseases. The training is executed in limited training dataset conditions. The numberof images per class ranges from a minimum of 30 to a maximum of 130. The accuracy and F1-score ofthe suggested architecture equal to 88.35% and 0.9 that is much better than pure transfer learning orold approach based on Triplet loss. New improved pipeline has been successfully implemented in theDoctorP platform, enhancing its ability to diagnose plant diseases with greater accuracy and reliability
ОИЯИ = ОИЯИ (JINR)2025
Спец.(статьи,препринты) = 28.0 - Биология$
Спец.(статьи,препринты) = Ц 849 - Искусственный интеллект. Теория и практика
Uzhinskiy, A.
Efficient Pipeline for Plant Disease Classification / A.Uzhinskiy. – Text: electronic // Natural Science Review. – 2025. – Vol. 2, No. 2. – P. 100201. – URL: https://nsr-jinr.ru/index.php/nsr/article/view/32/29. – Bibliogr.: 28.
Accurate identification of disease and correct treatment policy can save and increase yield. Differentdeep learning methods have emerged as an effective solution to this problem. Still, the challenges posedby limited datasets and the similarities in disease symptoms make traditional methods, such as transferlearning from models pre-trained on large-scale datasets like ImageNet, less effective. In this study, aself-collected dataset from the DoctorP project, consisting of 46 distinct classes and 2615 images, wasutilized. DoctorP is a multifunctional platform for plant disease detection oriented on agricultural andornamental crop. The platform has different interfaces like mobile applications for iOS and Android,a Telegram bot, and an API for external services. Users and services send photos of the diseasedplants in to the platform and can get prediction and treatment recommendation for their case. Theplatform supports a wide range of disease classification models. MobileNet_v2 and a Triplet lossfunction were previously used to create models. Extensive increase in the number of disease classesforces new experiment with architectures and training approaches. In the current research, an effectivesolution based on ConvNeXt architecture and Large Margin Cosine Loss is proposed to classify 46different plant diseases. The training is executed in limited training dataset conditions. The numberof images per class ranges from a minimum of 30 to a maximum of 130. The accuracy and F1-score ofthe suggested architecture equal to 88.35% and 0.9 that is much better than pure transfer learning orold approach based on Triplet loss. New improved pipeline has been successfully implemented in theDoctorP platform, enhancing its ability to diagnose plant diseases with greater accuracy and reliability
ОИЯИ = ОИЯИ (JINR)2025
Спец.(статьи,препринты) = 28.0 - Биология$
Спец.(статьи,препринты) = Ц 849 - Искусственный интеллект. Теория и практика