Поиск :
Личный кабинет :
Электронный каталог: Rusov, D. - Recurrent and Graph Neural Networks for Particle Tracking at the BM@N Experiment
Rusov, D. - Recurrent and Graph Neural Networks for Particle Tracking at the BM@N Experiment
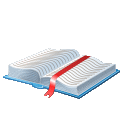
Книга (аналит. описание)
Автор: Rusov, D.
Advances in Neural Computation, Machine Learning, and Cognitive Research VI [Electronic resource]: Recurrent and Graph Neural Networks for Particle Tracking at the BM@N Experiment
б.г.
ISBN отсутствует
Автор: Rusov, D.
Advances in Neural Computation, Machine Learning, and Cognitive Research VI [Electronic resource]: Recurrent and Graph Neural Networks for Particle Tracking at the BM@N Experiment
б.г.
ISBN отсутствует
Книга (аналит. описание)
Rusov, D.
Recurrent and Graph Neural Networks for Particle Tracking at the BM@N Experiment / D.Rusov, P.Goncharov, E.Rezvaya, G.Ososkov, A.Zhemchugov, [a.o.] // Advances in Neural Computation, Machine Learning, and Cognitive Research VI [Electronic resource] : Selected Papers from the XXIV International Conference on Neuroinformatics, Moscow, Russia, October 17-21, 2022 / Ed.: B.Kryzhanovsky, W.Dunin-Barkowski, V.Redko, Y.Tiumentsev. – Cham : Springer, 2023. – P. 305-313. – URL: https://doi.org/10.1007/978-3-031-19032-2_32. – Bibliogr.: 12.
This work presents a new two-step approach for elementary particle tracking that combines the advantages of both local and global tracking algorithms. On the first stage, where the graph of possible track-candidates is too big to fit into memory, a recurrent neural network model TrackNETv3 is used for building track candidates. On the second stage there is a graph neural network GraphNet needed for clearing the graph from the fake segments. The results of testing the proposed approach on the 3,2 GeV Ar+Pb simulation for the BM@N RUN7 are presented. – (Studies in Computational Intelligence ; Vol. 1064) .
ОИЯИ = ОИЯИ (JINR)2023
Спец.(статьи,препринты) = Ц 84 а2 - Многомашинные комплексы вычислительных средств. Вычислительные системы и сети. Параллельные вычисления. Квантовые компьютеры
Спец.(статьи,препринты) = С 344.1т - Вопросы измерений в трековых камерах
Спец.(статьи,препринты) = С 325.1а - Нейронные сети и клеточные автоматы
Спец.(статьи,препринты) = Ц 849 - Искусственный интеллект. Теория и практика
Rusov, D.
Recurrent and Graph Neural Networks for Particle Tracking at the BM@N Experiment / D.Rusov, P.Goncharov, E.Rezvaya, G.Ososkov, A.Zhemchugov, [a.o.] // Advances in Neural Computation, Machine Learning, and Cognitive Research VI [Electronic resource] : Selected Papers from the XXIV International Conference on Neuroinformatics, Moscow, Russia, October 17-21, 2022 / Ed.: B.Kryzhanovsky, W.Dunin-Barkowski, V.Redko, Y.Tiumentsev. – Cham : Springer, 2023. – P. 305-313. – URL: https://doi.org/10.1007/978-3-031-19032-2_32. – Bibliogr.: 12.
This work presents a new two-step approach for elementary particle tracking that combines the advantages of both local and global tracking algorithms. On the first stage, where the graph of possible track-candidates is too big to fit into memory, a recurrent neural network model TrackNETv3 is used for building track candidates. On the second stage there is a graph neural network GraphNet needed for clearing the graph from the fake segments. The results of testing the proposed approach on the 3,2 GeV Ar+Pb simulation for the BM@N RUN7 are presented. – (Studies in Computational Intelligence ; Vol. 1064) .
ОИЯИ = ОИЯИ (JINR)2023
Спец.(статьи,препринты) = Ц 84 а2 - Многомашинные комплексы вычислительных средств. Вычислительные системы и сети. Параллельные вычисления. Квантовые компьютеры
Спец.(статьи,препринты) = С 344.1т - Вопросы измерений в трековых камерах
Спец.(статьи,препринты) = С 325.1а - Нейронные сети и клеточные автоматы
Спец.(статьи,препринты) = Ц 849 - Искусственный интеллект. Теория и практика