Поиск :
Личный кабинет :
Электронный каталог: Borisov, M. S. - Deep Learning Applications for Traffic Sign Detection and Classification
Borisov, M. S. - Deep Learning Applications for Traffic Sign Detection and Classification
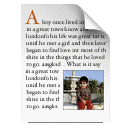
Статья
Автор: Borisov, M. S.
Физика элементарных частиц и атомного ядра. Письма: Deep Learning Applications for Traffic Sign Detection and Classification : [Abstract]
б.г.
ISBN отсутствует
Автор: Borisov, M. S.
Физика элементарных частиц и атомного ядра. Письма: Deep Learning Applications for Traffic Sign Detection and Classification : [Abstract]
б.г.
ISBN отсутствует
Статья
Borisov, M.S.
Deep Learning Applications for Traffic Sign Detection and Classification : [Abstract] / M.S.Borisov, G.A.Ososkov // Физика элементарных частиц и атомного ядра. Письма. – 2023. – Т.20, №5. – P.1352. – URL: http://www1.jinr.ru/Pepan_letters/panl_2023_5/71_Borisov_ann.pdf.
The paper addresses a new method proposed for detecting and identifying road infrastructure elements. The method can also be used for other image processing and analysis tasks. The method uses a YOLO-type single-stage visual detector to predict the coordinates of a certain number of bounding boxes with a MobileNet convolutional neural net used as a classifier. The tracking mechanism makes it possible to link the frames by assigning a unique number to every detected object. A large set of 160,000 traffic signs was used as the training data set for the model. A data flow architecture has been developed. The metrics generated and the rate of inference are sufficient to use as a model for collecting new data. The findings can be used for further analysis. The system is already being used in a road structure inventory project.
ОИЯИ = ОИЯИ (JINR)2023
Спец.(статьи,препринты) = Ц 843 - Распознавание образов
Спец.(статьи,препринты) = С 325.1а - Нейронные сети и клеточные автоматы
Borisov, M.S.
Deep Learning Applications for Traffic Sign Detection and Classification : [Abstract] / M.S.Borisov, G.A.Ososkov // Физика элементарных частиц и атомного ядра. Письма. – 2023. – Т.20, №5. – P.1352. – URL: http://www1.jinr.ru/Pepan_letters/panl_2023_5/71_Borisov_ann.pdf.
The paper addresses a new method proposed for detecting and identifying road infrastructure elements. The method can also be used for other image processing and analysis tasks. The method uses a YOLO-type single-stage visual detector to predict the coordinates of a certain number of bounding boxes with a MobileNet convolutional neural net used as a classifier. The tracking mechanism makes it possible to link the frames by assigning a unique number to every detected object. A large set of 160,000 traffic signs was used as the training data set for the model. A data flow architecture has been developed. The metrics generated and the rate of inference are sufficient to use as a model for collecting new data. The findings can be used for further analysis. The system is already being used in a road structure inventory project.
ОИЯИ = ОИЯИ (JINR)2023
Спец.(статьи,препринты) = Ц 843 - Распознавание образов
Спец.(статьи,препринты) = С 325.1а - Нейронные сети и клеточные автоматы