Поиск :
Личный кабинет :
Электронный каталог: Filippatos, G. - Machine Learning for the EUSO-SPB2 Fluorescence Telescope Data Analysis
Filippatos, G. - Machine Learning for the EUSO-SPB2 Fluorescence Telescope Data Analysis
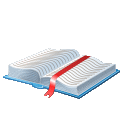
Книга (аналит. описание)
Автор: Filippatos, G.
38th International Cosmic Ray Conference (ICRC2023), Nagoya, Japan, 26 July - 3 August, 2023 [Electronic resource]: Machine Learning for the EUSO-SPB2 Fluorescence Telescope Data Analysis
б.г.
ISBN отсутствует
Автор: Filippatos, G.
38th International Cosmic Ray Conference (ICRC2023), Nagoya, Japan, 26 July - 3 August, 2023 [Electronic resource]: Machine Learning for the EUSO-SPB2 Fluorescence Telescope Data Analysis
б.г.
ISBN отсутствует
Книга (аналит. описание)
Filippatos, G.
Machine Learning for the EUSO-SPB2 Fluorescence Telescope Data Analysis / G.Filippatos, M.Zotov, D.Naumov, L.G.Tkachev, [a.o.] // 38th International Cosmic Ray Conference (ICRC2023), Nagoya, Japan, 26 July - 3 August, 2023 [Electronic resource]. – Trieste : SISSA, 2024. – P. 234. – URL: https://doi.org/10.22323/1.444.0234. – Bibliogr.: 18.
The Extreme Universe Space Observatory on a Super Pressure Balloon 2 (EUSO-SPB2) is the most advanced balloon mission undertaken by the JEM-EUSO collaboration. EUSO-SPB2 is built on the experience of previous stratosphere missions, EUSO-Balloon and EUSO-SPB, and of the Mini-EUSO space mission currently active onboard the International Space Station. EUSO- SPB2 is equipped with two instruments: a fluorescence telescope aimed at registering ultra-high energy cosmic rays (UHECRs) with an energy above 2 EeV and a Cherenkov telescope built to measure direct Cherenkov emission from cosmic rays with energies above 1 PeV. The EUSO-SPB2 mission will provide pioneering observations on the path towards a space-based multi-messenger observatory. As such, a special attention was paid to the development of triggers and other software aimed at comprehensive data analysis. A whole number of methods based on machine learning (ML) and neural networks was developed during the construction of the experiment and a few others are under active development. Here we provide a brief review of the ML-based methods already implemented in the instrument and the ground software and report preliminary results on the ML-based reconstruction of UHECR parameters for the fluorescence telescope – (Proceedings of Science ; Vol. 444) .
ОИЯИ = ОИЯИ (JINR)2024
Спец.(статьи,препринты) = С 347 - Космические лучи
Спец.(статьи,препринты) = Ц 849 - Искусственный интеллект. Теория и практика
Спец.(статьи,препринты) = Ц 840 в - Программы обработки экспериментальных данных и управление физическими установками$
Спец.(статьи,препринты) = С 63 - Астрофизика$
Filippatos, G.
Machine Learning for the EUSO-SPB2 Fluorescence Telescope Data Analysis / G.Filippatos, M.Zotov, D.Naumov, L.G.Tkachev, [a.o.] // 38th International Cosmic Ray Conference (ICRC2023), Nagoya, Japan, 26 July - 3 August, 2023 [Electronic resource]. – Trieste : SISSA, 2024. – P. 234. – URL: https://doi.org/10.22323/1.444.0234. – Bibliogr.: 18.
The Extreme Universe Space Observatory on a Super Pressure Balloon 2 (EUSO-SPB2) is the most advanced balloon mission undertaken by the JEM-EUSO collaboration. EUSO-SPB2 is built on the experience of previous stratosphere missions, EUSO-Balloon and EUSO-SPB, and of the Mini-EUSO space mission currently active onboard the International Space Station. EUSO- SPB2 is equipped with two instruments: a fluorescence telescope aimed at registering ultra-high energy cosmic rays (UHECRs) with an energy above 2 EeV and a Cherenkov telescope built to measure direct Cherenkov emission from cosmic rays with energies above 1 PeV. The EUSO-SPB2 mission will provide pioneering observations on the path towards a space-based multi-messenger observatory. As such, a special attention was paid to the development of triggers and other software aimed at comprehensive data analysis. A whole number of methods based on machine learning (ML) and neural networks was developed during the construction of the experiment and a few others are under active development. Here we provide a brief review of the ML-based methods already implemented in the instrument and the ground software and report preliminary results on the ML-based reconstruction of UHECR parameters for the fluorescence telescope – (Proceedings of Science ; Vol. 444) .
ОИЯИ = ОИЯИ (JINR)2024
Спец.(статьи,препринты) = С 347 - Космические лучи
Спец.(статьи,препринты) = Ц 849 - Искусственный интеллект. Теория и практика
Спец.(статьи,препринты) = Ц 840 в - Программы обработки экспериментальных данных и управление физическими установками$
Спец.(статьи,препринты) = С 63 - Астрофизика$