Поиск :
Личный кабинет :
Электронный каталог: Cortina Gil, E. - Improved Calorimetric Particle Identification in NA62 Using Machine Learning Techniques
Cortina Gil, E. - Improved Calorimetric Particle Identification in NA62 Using Machine Learning Techniques
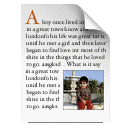
Статья
Автор: Cortina Gil, E.
Journal of High Energy Physics [Electronic resource]: Improved Calorimetric Particle Identification in NA62 Using Machine Learning Techniques
б.г.
ISBN отсутствует
Автор: Cortina Gil, E.
Journal of High Energy Physics [Electronic resource]: Improved Calorimetric Particle Identification in NA62 Using Machine Learning Techniques
б.г.
ISBN отсутствует
Статья
Cortina Gil, E.
Improved Calorimetric Particle Identification in NA62 Using Machine Learning Techniques / E.Cortina Gil, A.Baeva, D.Baigarashev, D.Emelyanov, T.Enik, V.Falaleev, V.Kekelidze, D.Kereibay, A.Korotkova, L.Litov, D.Madigozhin, M.Misheva, N.Molokanova, S.Movchan, I.Polenkevich, Yu.Potrebenikov, S.Shkarovskiy, A.Zinchenko, [NA62 Collab.] // Journal of High Energy Physics [Electronic resource]. – 2023. – Vol.2023, No.11. – P. 138. – URL: https://doi.org/10.1007/JHEP11(2023)138. – Bibliogr.: 19.
Measurement of the ultra-rare K+ → π+ν ¯ν decay at the NA62 experiment at CERN requires high-performance particle identification to distinguish muons from pions. Calorimetric identification currently in use, based on a boosted decision tree algorithm, achieves a muon misidentification probability of 1.2 × 10*-*5 for a pion identification efficiency of 75% in the momentum range of 15–40 GeV/c. In this work, calorimetric identification performance is improved by developing an algorithm based on a convolutional neural network classifier augmented by a filter. Muon misidentification probability is reduced by a factor of six with respect to the current value for a fixed pion-identification efficiency of 75%. Alternatively, pion identification efficiency is improved from 72% to 91% for a fixed muon misidentification probability of 10*-*5.
ОИЯИ = ОИЯИ (JINR)2023
Спец.(статьи,препринты) = С 346.5б - Свойства К-мезонов: масса, спин, четность, схемы и времена распадов
Спец.(статьи,препринты) = Ц 849 - Искусственный интеллект. Теория и практика
Cortina Gil, E.
Improved Calorimetric Particle Identification in NA62 Using Machine Learning Techniques / E.Cortina Gil, A.Baeva, D.Baigarashev, D.Emelyanov, T.Enik, V.Falaleev, V.Kekelidze, D.Kereibay, A.Korotkova, L.Litov, D.Madigozhin, M.Misheva, N.Molokanova, S.Movchan, I.Polenkevich, Yu.Potrebenikov, S.Shkarovskiy, A.Zinchenko, [NA62 Collab.] // Journal of High Energy Physics [Electronic resource]. – 2023. – Vol.2023, No.11. – P. 138. – URL: https://doi.org/10.1007/JHEP11(2023)138. – Bibliogr.: 19.
Measurement of the ultra-rare K+ → π+ν ¯ν decay at the NA62 experiment at CERN requires high-performance particle identification to distinguish muons from pions. Calorimetric identification currently in use, based on a boosted decision tree algorithm, achieves a muon misidentification probability of 1.2 × 10*-*5 for a pion identification efficiency of 75% in the momentum range of 15–40 GeV/c. In this work, calorimetric identification performance is improved by developing an algorithm based on a convolutional neural network classifier augmented by a filter. Muon misidentification probability is reduced by a factor of six with respect to the current value for a fixed pion-identification efficiency of 75%. Alternatively, pion identification efficiency is improved from 72% to 91% for a fixed muon misidentification probability of 10*-*5.
ОИЯИ = ОИЯИ (JINR)2023
Спец.(статьи,препринты) = С 346.5б - Свойства К-мезонов: масса, спин, четность, схемы и времена распадов
Спец.(статьи,препринты) = Ц 849 - Искусственный интеллект. Теория и практика